Elevating fashion e-commerce with contextual intelligence
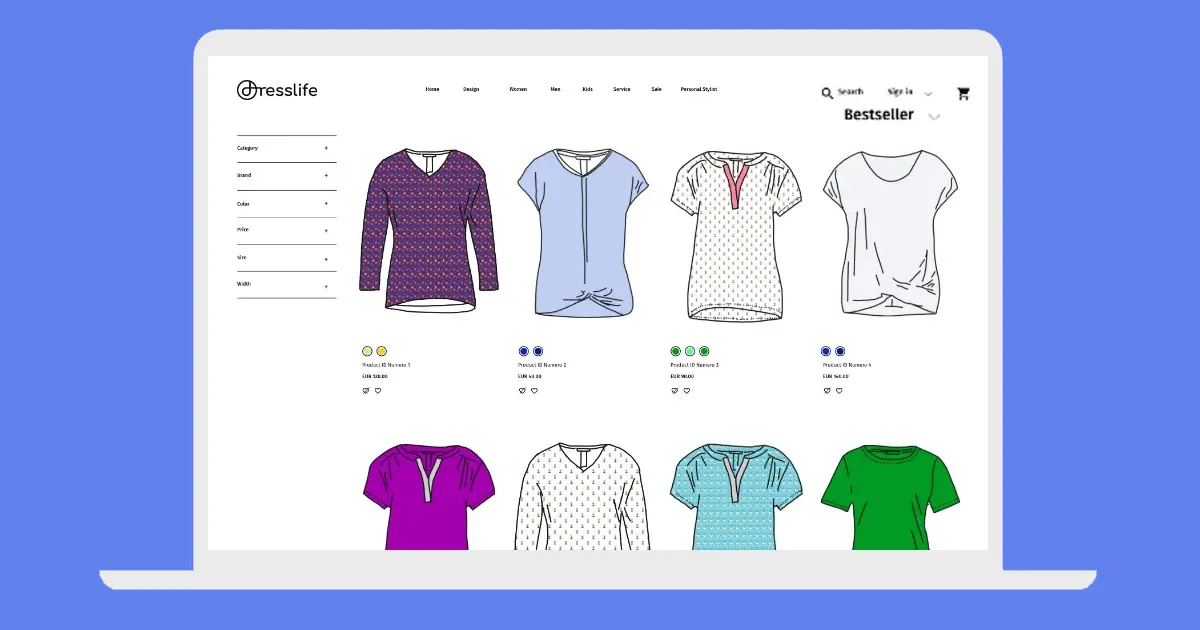
We're excited to continue our long-term partnership with Hannover University's TNT Institute by enhancing AI-driven fashion recommendations with Contextual Multi-Armed Bandits (MABs). This is our third research project co-funded by the EU and the state of Lower Saxony.
Exploring MABs
Imagine an AI with the skill of a seasoned gambler, adept at choosing the winning slot machine, consistently maximizing profits. That's the role of a Multi-Armed Bandit (MAB) – a self-learning algorithm expertly balancing efforts across various options. In fashion e-commerce, recommendation engines can act as personal assistants, tailoring clothing selections to individual tastes . The Multi-Armed Bandit (MAB) steps in as the savvy manager, perpetually selecting the ideal assistants for each customer, ensuring customers are always presented with the most appealing fashion choices.
Maximizing Profitability
MABs are essential for addressing significant shifts in fashion buying behavior, particularly during peak seasons like Black Week. AI effectiveness depends on the data it's trained on. AIs trained on limited data may not work during unique events like Black Friday. MABs, that pick AIs optimized for such occasions, ensure our recommendations are effective and revenue is maximized at crucial times. The next step, Contextual Multi-Armed Bandits, promises even greater precision by tailoring recommendations to the specific context of each customer.
To enhance your gross profit per user after returns, connect with us.